Overview
Subscribe to Our Newsletter
Get expert guidance on various topics, resources, and exclusive insights
Top 5 Use Cases for Natural Language Processing in Healthcare
In healthcare, information is only valuable when it is accessible, structured, and ready to act on. The industry generates massive volumes of unstructured data on a daily basis, from handwritten clinical notes to discharge summaries and patient histories.
Turning this data into meaningful insights is a major challenge, and NLP is one of the most effective solutions equipped to do exactly that.
At Veroke, we’ve experienced the transformative impact of NLP firsthand.
From developing solutions that extract insights from large historical datasets to building systems that convert natural language prompts into structured outputs, we have worked with NLP across a range of real-world applications.
These experiences have shown us how NLP can simplify complexity and turn data into usable insights, and provide value. And the healthcare sector is quickly catching on.
In 2023, the global NLP market in healthcare and life sciences was valued at $4.9 billion, and it’s just getting started, with forecasts projecting a staggering CAGR of 34.7% through 2030.
Behind this rapid growth is a shared realization: NLP is no longer experimental; it’s becoming imperative to how modern healthcare operates.
But what does that look like in practice? Let’s explore the top five use cases where NLP is already making a measurable difference across healthcare.
Understanding Natural Language Processing
Simply put, Natural Language Processing (NLP) is a specialized branch of Artificial Intelligence that enables computers to understand, interpret, and process unstructured human language.
Using machine learning algorithms, linguistic rules, and deep learning models, it identifies patterns in human communication and transforms them into actionable insights.
NLP proves beneficial for many industries through its ability to analyze unstructured data, automate repetitive tasks, and enable real-time insights.
From enhancing customer experiences in retail to improving decision-making in finance, NLP has transformed how organizations process and utilize information.
So, considering its applications and the potential it brings, we decided to explore what it offers to the healthcare industry, because it can help unlock the value from unstructured data.
NLP in Healthcare: Why It Matters?
Before we jump into the use cases, let’s first answer a fundamental question: What does NLP do in the health sector?
It enables computers to comprehend, interpret, and even generate human language. It plays a key role in transforming unstructured data, like clinical notes, patient histories, and lab reports, into structured, searchable, and usable information.
This empowers healthcare companies to quickly extract insights and make informed decisions, and opens the door to more efficient, data-driven healthcare services, without getting buried under mountains of paperwork.
Challenges That NLP Solves
The healthcare industry generates a massive amount of data every day. And this data often goes underutilized due to the complexity and format of healthcare technology used to capture it.
Traditional methods of data entry are not only time-consuming but also prone to human error. These can lead to delayed diagnoses, miscommunication, and even compromised patient care.
NLP addresses these challenges head-on by:
- Automating data extraction from clinical texts
- Improving documentation accuracy
- Reducing the administrative burden on healthcare professionals
- Accelerating access to critical patient information
How Does NLP Work in Healthcare?
NLP systems go beyond simply reading words; they are capable of understanding the context. These systems analyze medical documents by recognizing not just individual characters and phrases, but the exact meaning behind them.
For instance, it can distinguish between a patient’s name, a symptom, a diagnosis, or even determine if the information refers to multiple patients within a single record.
Once the input is processed, NLP applies a mix of AI techniques, like using deep learning, rule-based models, and semantic analysis, to extract relevant data and format it as needed.
It means it can identify and segment crucial information such as patient IDs, prescribed medications, lab results, and clinical symptoms, then map them to the correct fields within Electronic Health Record (EHR) systems.
Another important aspect here that makes it even more powerful in healthcare is its ability to learn and improve over time.
Because its advanced models, trained on large volumes of medical data, continuously refine their accuracy, becoming smarter and more efficient at interpreting complex information.
The Impact of NLP in Healthcare: Benefits That Matter
The integration of NLP in healthcare offers a multitude of advantages for providers, administrators, and researchers alike.
As healthcare companies rely on technology and data-driven approaches these days, NLP becomes essential in unlocking the value of unstructured data and driving operational efficiency.
⤷ Here are some of the most impactful benefits NLP brings to the table:
1. Optimized Clinical Documentation
With an estimated 80% of healthcare data being unstructured, extracting key insights from medical records is both tedious and error-prone.
NLP automates this process, accurately pulling relevant information from handwritten or transcribed documents. And the result? Faster, cleaner, and more reliable documentation that forms the backbone of effective patient care.
2. Enhanced Patient Care and Diagnosis
When administrative workloads are reduced, physicians can spend more time with their patients.
NLP supports this by automating data entry and surfacing critical insights from medical texts, allowing clinicians to focus on timely diagnoses and treatment plans. With better access to patient data, providers can make more informed decisions, improving quality of care across the board.
3. Efficient Data Extraction and Analysis
From analyzing clinical trial reports to summarizing vast amounts of medical literature, NLP-powered systems can process and interpret large datasets with speed and precision.
This helps researchers and clinicians identify trends, correlations, and evidence-based insights, accelerating medical innovation and improving patient outcomes.
4. Streamlined Administrative Processes
Manual documentation and data management tasks are a significant drain on healthcare staff.
NLP reduces this burden by automating repetitive processes such as transcribing notes, updating patient records, and coding for billing. By minimizing administrative strain, healthcare teams can direct more attention to patient engagement and clinical excellence.
Natural Language Processing Techniques in Healthcare
Before we get into the topic of exploring the use cases in healthcare that technology brings, it’s important to understand the key techniques that power this transformation.
These core NLP methods are the building blocks behind intelligent systems that can interpret and act on human language in medical contexts.
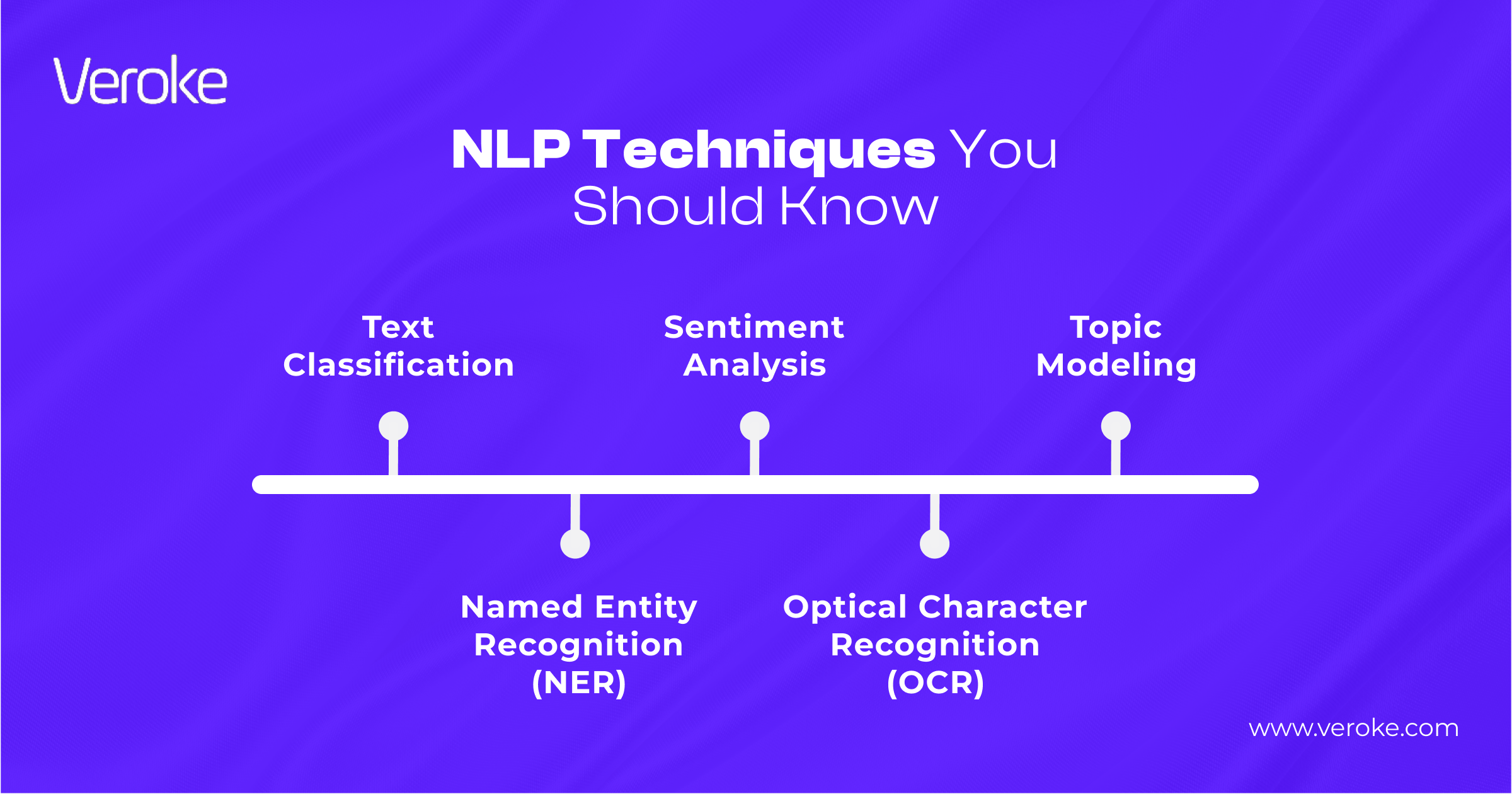
5 Key NLP Techniques in Healthcare
1. Optical Character Recognition (OCR)
OCR enables computers to “read” printed or handwritten text and convert it into digital formats. This means scanning patient intake forms, clinical notes, discharge summaries, and test results to turn paper-based or image-based information into structured data.
Once digitized, this data can seamlessly flow into an NLP pipeline for further analysis and integration with Electronic Health Records (EHRs).
2. Named Entity Recognition (NER)
NER is a powerful technique used to identify and classify key pieces of information, like patient names, location, drug names, or organization, into predefined categories.
In healthcare, NER can extract vital elements from complex clinical notes or lab reports and link them to structured medical databases.
3. Sentiment Analysis
Sentiment analysis evaluates the emotional tone behind a body of text. While often used in marketing to gauge customer feedback, it’s also gaining relevance in healthcare.
For instance, providers can assess patient reviews or feedback from social media to understand overall satisfaction, identify areas of concern, and refine the patient experience.
4. Text Classification
Text classification, also known as text categorization, this technique involves assigning tags or labels to text based on its content.
It can help flag high-risk patients, categorize medical conditions, or prioritize cases by urgency, just by analyzing keywords or phrases found in clinical records or notes.
5. Topic Modeling
Topic modeling groups documents together based on shared themes or semantic patterns. Using statistical models like Latent Dirichlet Allocation (LDA), it can uncover hidden topics in large datasets.
For instance, identifying frequently discussed symptoms in medical research or clustering patient complaints into common categories for quality improvement.
Top 5 Use Cases of NLP in the Healthcare Industry
Let’s dive into five key use cases where NLP is driving transformation in the healthcare sector.
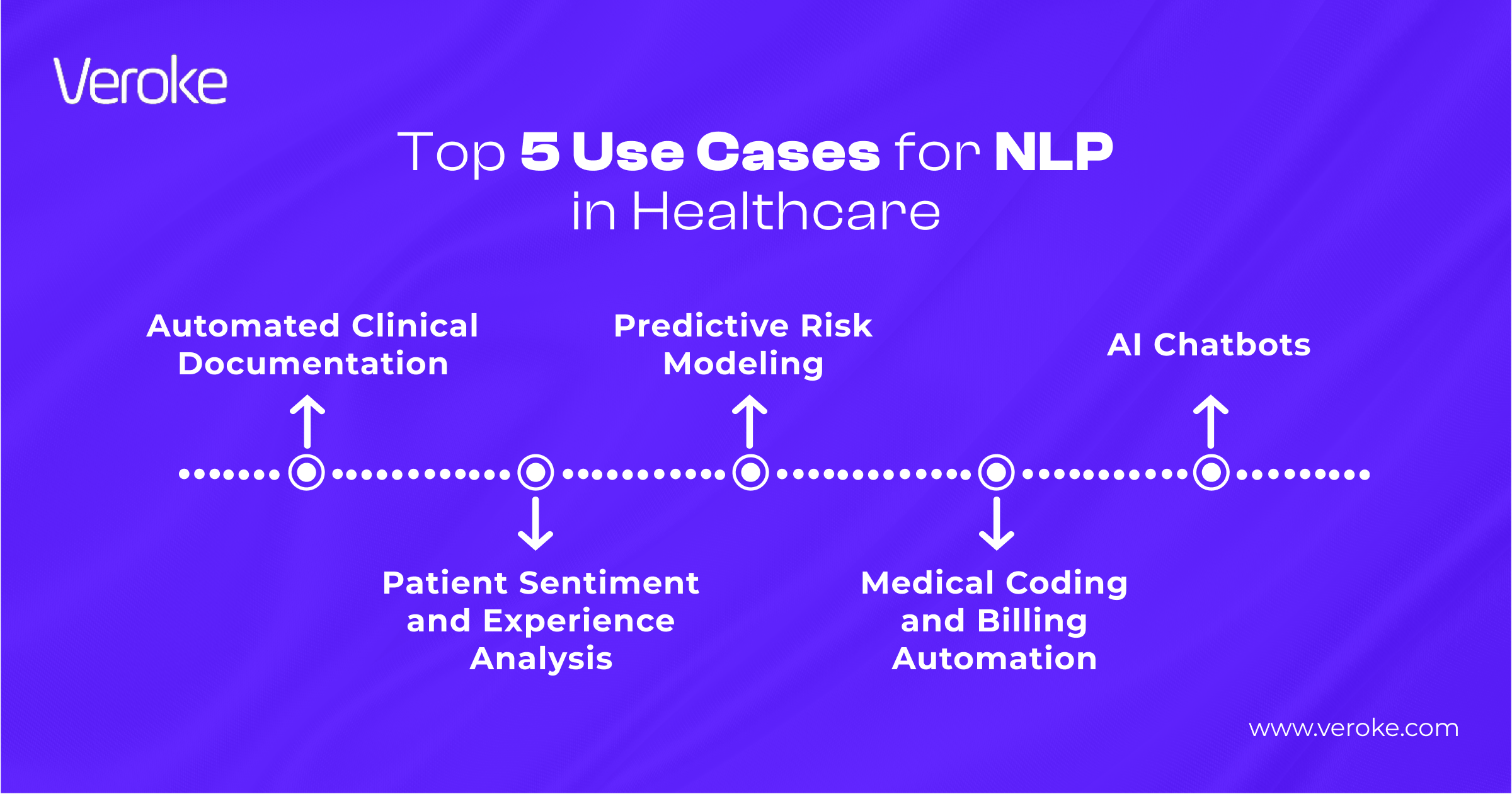
Natural Language Processing Key Use Cases
1. Automated Clinical Documentation
One of the most time-consuming aspects of healthcare is the documentation of patient encounters. Normally, physicians spend a substantial portion of their day typing notes or dictating information to be transcribed.
This process is not only slow but can also result in inaccurate records due to human error or misinterpretation. NLP addresses this challenge by automating clinical documentation, allowing healthcare professionals to capture critical patient data in real time.
NLP tools can automatically transcribe physician-patient conversations, extract relevant data from clinical notes, and even summarize key findings. By feeding this information directly into Electronic Health Records (EHRs), NLP ensures that patient information is up to date and accessible without requiring manual input.
2. Predictive Risk Modeling
One of the most significant challenges in healthcare is predicting which patients are at risk for certain conditions before symptoms become severe. By analyzing unstructured data from clinical notes, lab reports, medical histories, and physician notes, NLP can identify patterns and risk factors that may otherwise go unnoticed.
This predictive power allows healthcare providers to intervene early, often preventing costly and life-threatening complications. NLP-enhanced predictive models can process a vast array of medical data, looking for subtle correlations and early warning signs.
It can flag early indicators of conditions like sepsis, heart failure, or mental health issues, providing physicians with critical information that might lead to earlier, more effective interventions.
3. Medical Coding and Billing Automation
Accurate medical coding is critical for insurance claims and proper reimbursement, but it is often a complicated and manual process. Healthcare providers rely on coding specialists to assign the correct codes to diagnoses and procedures, but errors in coding can lead to rejected claims, delayed reimbursements, or even legal issues.
NLP automates medical coding by analyzing clinical documentation and assigning the correct codes based on the content. By automating, healthcare organizations can reduce human error and streamline their revenue cycle.
This not only speeds up the billing process but also ensures that claims are more accurate, which leads to faster payments and fewer claim rejections. Not only that, by freeing up coding specialists to focus on more complex cases, it improves operational efficiency across the entire healthcare system.
4. Patient Sentiment and Experience Analysis
Understanding patient sentiment and experience is crucial for improving the quality of care and maintaining a positive reputation. With NLP, you can analyze a broader range of patient feedback, including online reviews, social media posts, and comments from patient surveys.
NLP tools can automatically analyze this data to extract valuable insights about patient perceptions of care, hospital services, and treatment outcomes.
By processing thousands of patient comments, NLP can identify common themes, pinpoint recurring issues, and provide actionable recommendations for improving the patient experience. This enables healthcare organizations to respond quickly to patient concerns, enhance satisfaction, and drive continuous improvement.
5. AI Chatbots
NLP-powered chatbots are playing an increasingly vital role in modern healthcare, offering practical solutions to streamline patient interactions. The chatbots equipped with natural language understanding can handle a range of patient-facing tasks, such as answering FAQs, collecting symptom information, booking appointments, and offering medication reminders.
These digital assistants are available 24/7, providing patients with immediate support and reducing the load on front-desk staff.
Beyond just efficiency, these tools are also enhancing the patient experience. By minimizing wait times and delivering quick, accurate responses, NLP chatbots create a more connected and responsive healthcare environment. In terms of value, these technologies are cost-effective, scalable, and widely accessible.
Why Veroke Believes NLP Can Transform Healthcare?
At Veroke, we’ve worked on several projects that demonstrate the real-world potential of NLP in the health sector. Across different domains, we’ve seen how NLP can simplify complex data, automate insight extraction, and turn unstructured information into meaningful intelligence.
One of our AI-powered solutions involves generating complete user interfaces from natural language prompts. This tool transforms high-level ideas into deployable front-ends, a capability that could accelerate the development of healthcare applications, from patient portals to internal dashboards.
In another initiative, we built a document analysis platform to process large historical archives. Using NLP, we summarized lengthy documents, extracted key entities, and visualized insights through word clouds.
This same approach can be applied to clinical records, helping streamline medical research, case reviews, and compliance processes. This project explored how combining NLP with computer vision, using models like Florence 2, can assist in disease-related image analysis, such as identifying visual indicators of skin conditions like cancer or allergies.
While each project tackles a different challenge, the impact is consistent. NLP brings clarity to complexity, speed to workflows, and intelligence to every interaction. In a data-heavy industry like healthcare, these advantages can drive real improvements in care delivery, research, and operational efficiency.
Summing Up
As the healthcare industry undergoes rapid digital transformation, NLP is proving to be a game-changer. From streamlining data management to automating routine workflows, it doesn’t just enhance efficiency, it elevates the entire patient experience.
At its core, NLP empowers healthcare professionals with timely, data-driven insights. That means faster decisions, more accurate diagnoses, and ultimately, better patient outcomes.
At Veroke, we build AI-powered solutions that solve real-world healthcare challenges. Whether you’re looking to automate medication delivery, enhance patient engagement, or extract deep insights from unstructured medical data, we’ve got you covered.
Backed by a team of industry experts, we’re here to help you harness the full potential of AI in healthcare. Contact us today and let’s build the future together.
FAQs
1. How can NLP be used in healthcare?
Some clinical documents, like patient reports, need to follow a structured format. NLP helps by allowing healthcare professionals to write freely in an unstructured, narrative style, then automatically edits and organizes the content into the required structured format.
2. What is the use case of NLP in healthcare with ethical considerations?
NLP streamlines clinical documentation by automating the process. Advanced healthcare-focused NLP tools can transcribe and interpret clinician-patient conversations, transforming unstructured speech or text into structured data, greatly reducing the documentation burden on physicians and saving them valuable time.
3. What is NLP with an example?
Unsupervised NLP relies on statistical language models to identify patterns in non-labeled input data. A common example is the autocomplete feature in messaging apps, which predicts contextually appropriate words by analyzing and learning from user input without needing predefined labels.
Transform your Ideas into a Digital Reality
Get customized bespoke
solutions for your business.
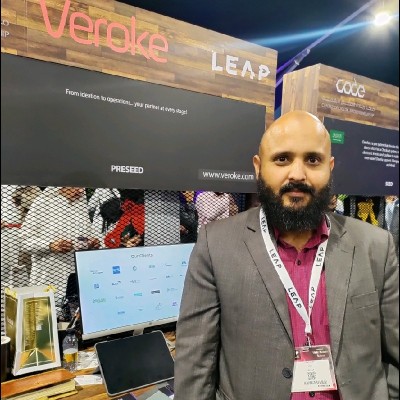